No Tradeoffs: Responsive Improves Kafka Streams on Every Dimension, Including Cost
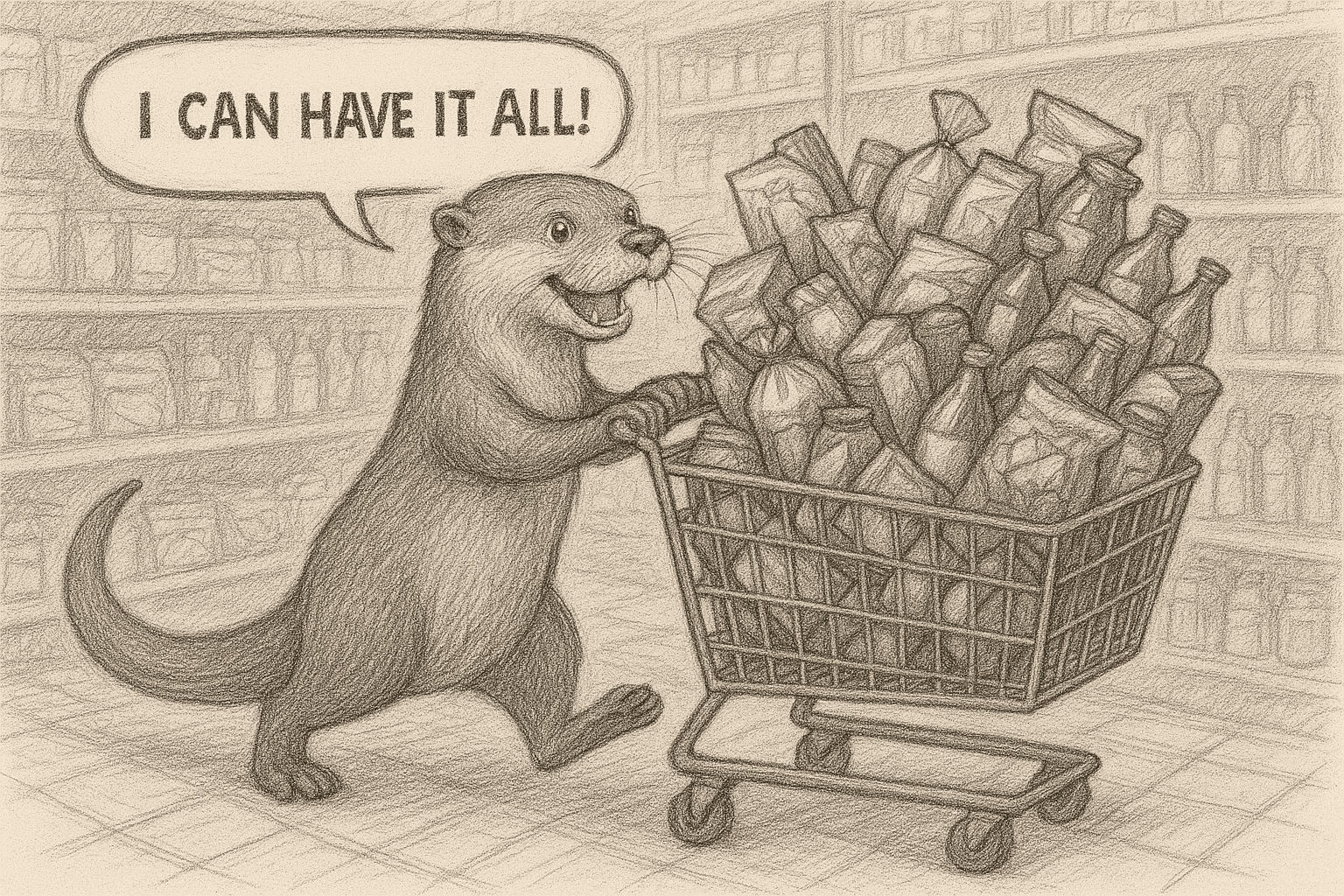
I am certainly biased, but I think it makes no sense to run Kafka Streams without Responsive. The migration cost is nearly zero — we are 100% compatible with the open source API. The reliability is 10x better and there is no tradeoff to performance. Honestly, it’s a no-brainer… until you worry about additional spend.
Why would you pay us something, when you don’t pay anything for Kafka Streams today?
The truth is, Kafka Streams is already on your bill — just hidden under compute, storage, and Kafka costs. Those costs can add up faster than you’d expect.
But even if you properly account for Kafka Streams’ hidden costs, Responsive could still come out more expensive when you factor in the need for a separate OLTP database.
I don’t want developers building realtime systems to choose between reliability and scalability on one hand, and cost-efficiency on the other. I want you to have it all—because that’s the only way Kafka Streams can win in this increasingly competitive space.
We’ve been working hard to make that possible. And now, with autoscaling, a new extremely cost-efficient database (RS3) and our innovative pricing model, I’m confident Responsive customers no longer need to choose between resilience and cost.
TL;DR - Look, you can read this post to convince yourself that Responsive cuts your infra spend on Kafka Streams. Or, just try it out yourself and see.
How Expensive is Kafka Streams without Responsive?
I’ll be blunt. I spent dozens of hours researching and building https://www.kafkastreams.money/ to convince myself (and now that I’m convinced, to convince you) that Responsive is no more expensive than running Kafka Streams without us. Instead, I discovered that it can be significantly cheaper.
Consider this open source application (it’s also the default configuration on the calculator, so feel free to play around with the numbers yourself if you want). There are a few important details to note:
- The application processes 3K events/s during the daytime (US) but only 1K events/s during the night and weekends.
- It typically needs to perform a full restoration 3/4 times a month. This happens during some deployments and due to the occasional incidents that triggers a rebalance. It has a 400GB changelog topic.
- It must be highly available, so its deployed with standby replicas.
Assuming it runs with 2 m5.xlarge instances, I’ve copied the breakdown of the costs here below:
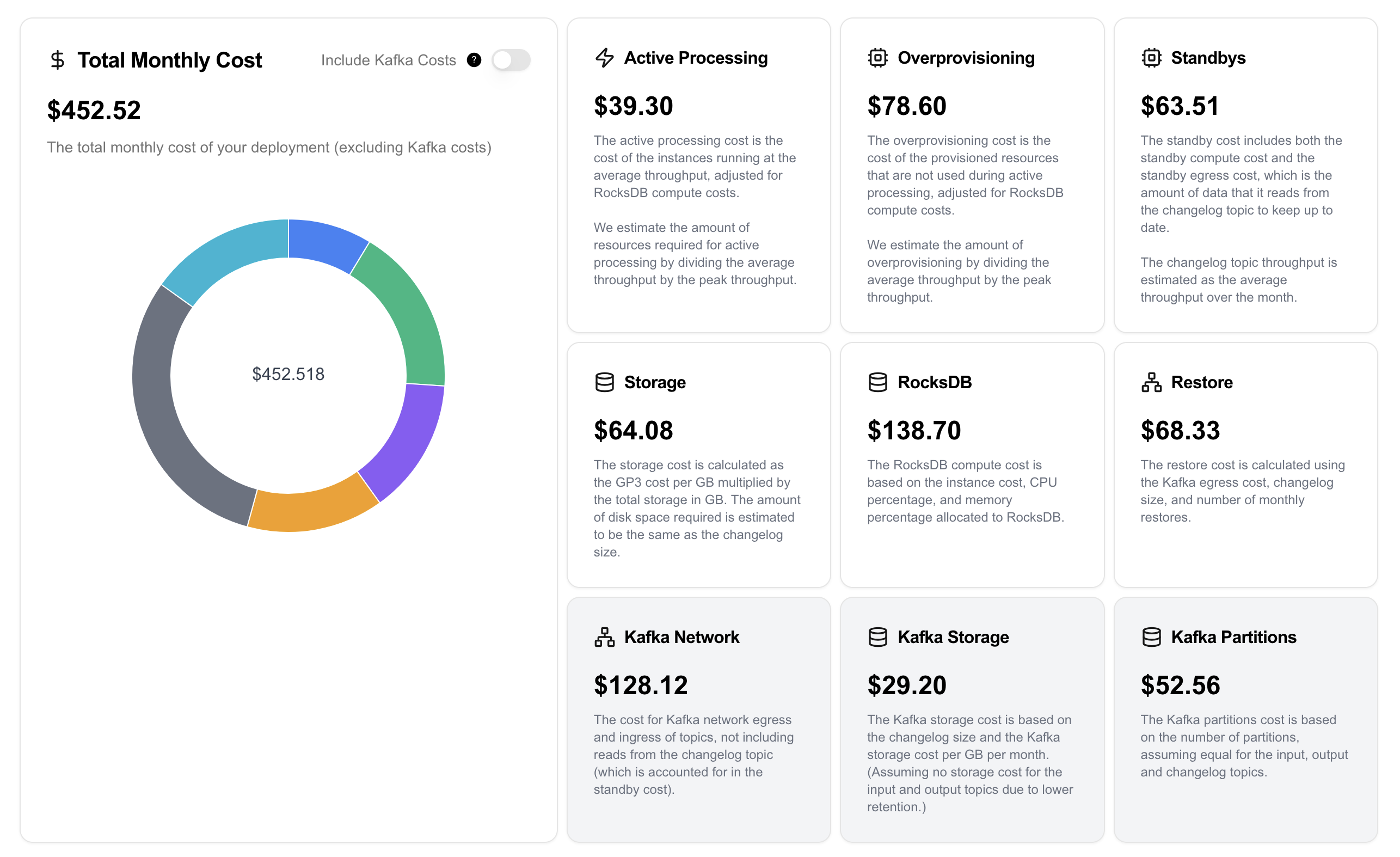
Active Processing is 10% of your Total Cost
When you run Kafka Streams, you aren’t paying to process events — you’re paying to store them, replicate them, restore them, and make sure you can handle bursts of them.
What’s worse, most of the time you have idle CPU and under-utilized memory because you must provision stateful applications for peak or risk instability with restores and rebalances.
It’s pretty common for an application workload to look something like this, where during weekends and nights there’s significantly less traffic than the daytime:
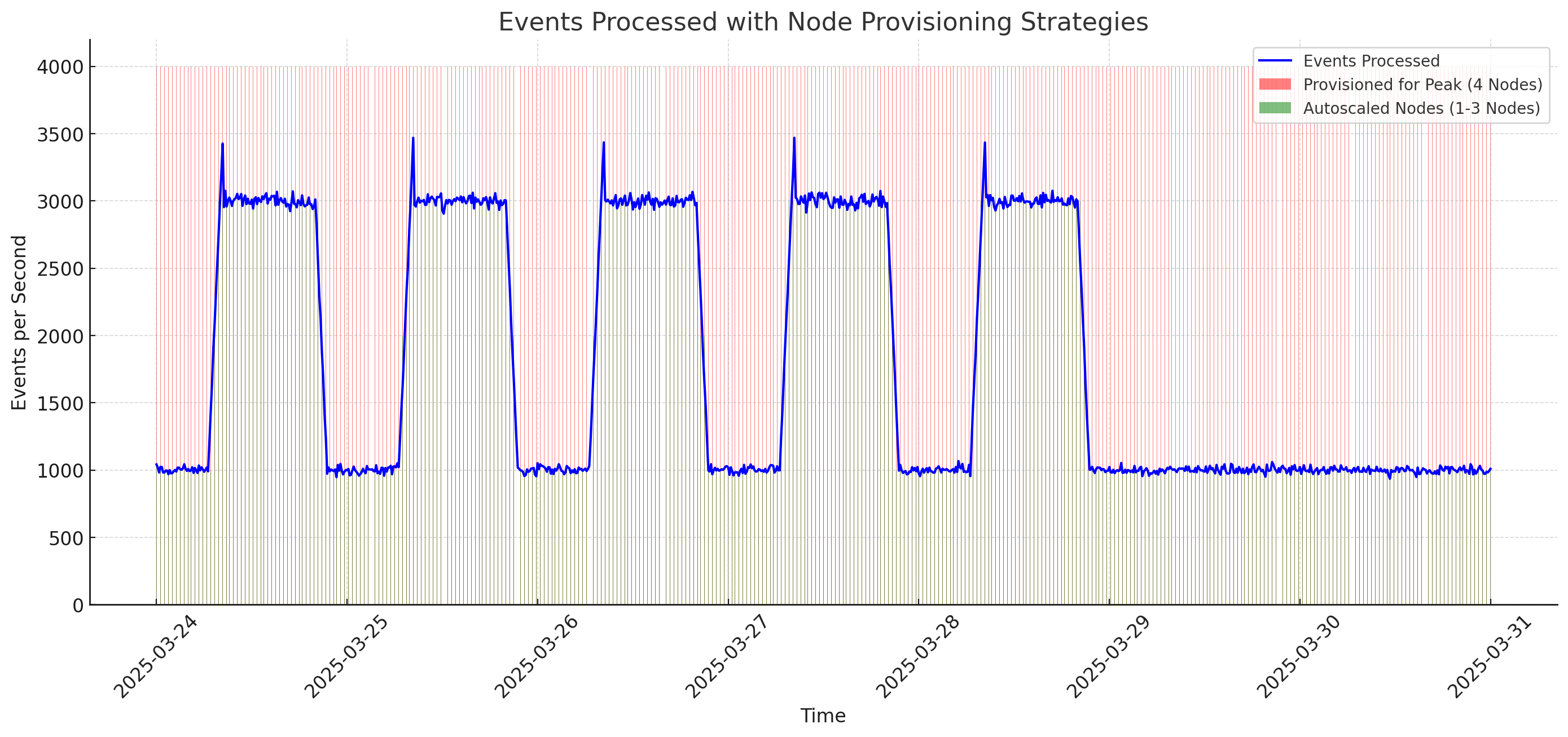
In this situation, you likely need to provision enough capacity to process ~4000 events per second to account for a potential burst in traffic. The Kafka Streams calculator takes this into account, and the part of your compute allocated to processing events is split into two categories: active processing and over-provisioning.
The conclusion here is clear: properly sized, autoscaled clusters can significantly cut your required compute for serving a stateful application.
RocksDB is Far From Free
Yes, it’s embedded. Yes, it’s pretty efficient. That doesn’t mean it’s free.
First, you’re paying for something like EBS storage (which we recommend over something like NVMe since that would risk significant outages) and potentially provisioned IOPS to meet your workload’s demands. And while EBS is relatively cheap, RocksDB gives you no option other than to store your entire dataset on it, which is still about 4x more expensive than S3.
Next, you can roughly assume that about 50% of your instance memory and 25% of your compute are dedicated to RocksDB. The memory is necessary for the caching to be effective and the CPU is necessary for compactions.
But what’s worse is that when RocksDB is coupled with your stream processing you’re forced to scale both, independent of which one is the bottleneck. Even if you could autoscale, you’re still wasting resources.
For example, let’s assume that the cardinality of the keyspace doesn’t change much whether I’m processing 1k events/s or 4k events/s (this means that the same keys are just getting more updates, as opposed to more keys being updated). In this situation, I may be fine with the same amount of RocksDB cache memory in both scenarios.
Unfortunately, if I’ve coupled RocksDB with my Stream Processor, I will likely need more CPU to handle the de/serialization of those 4k events/s. This forces me to scale up, but I’ve already configured my JVM size relative to my total container available memory so with every additional container I allocate I’m allocating unnecessary memory to RocksDB.
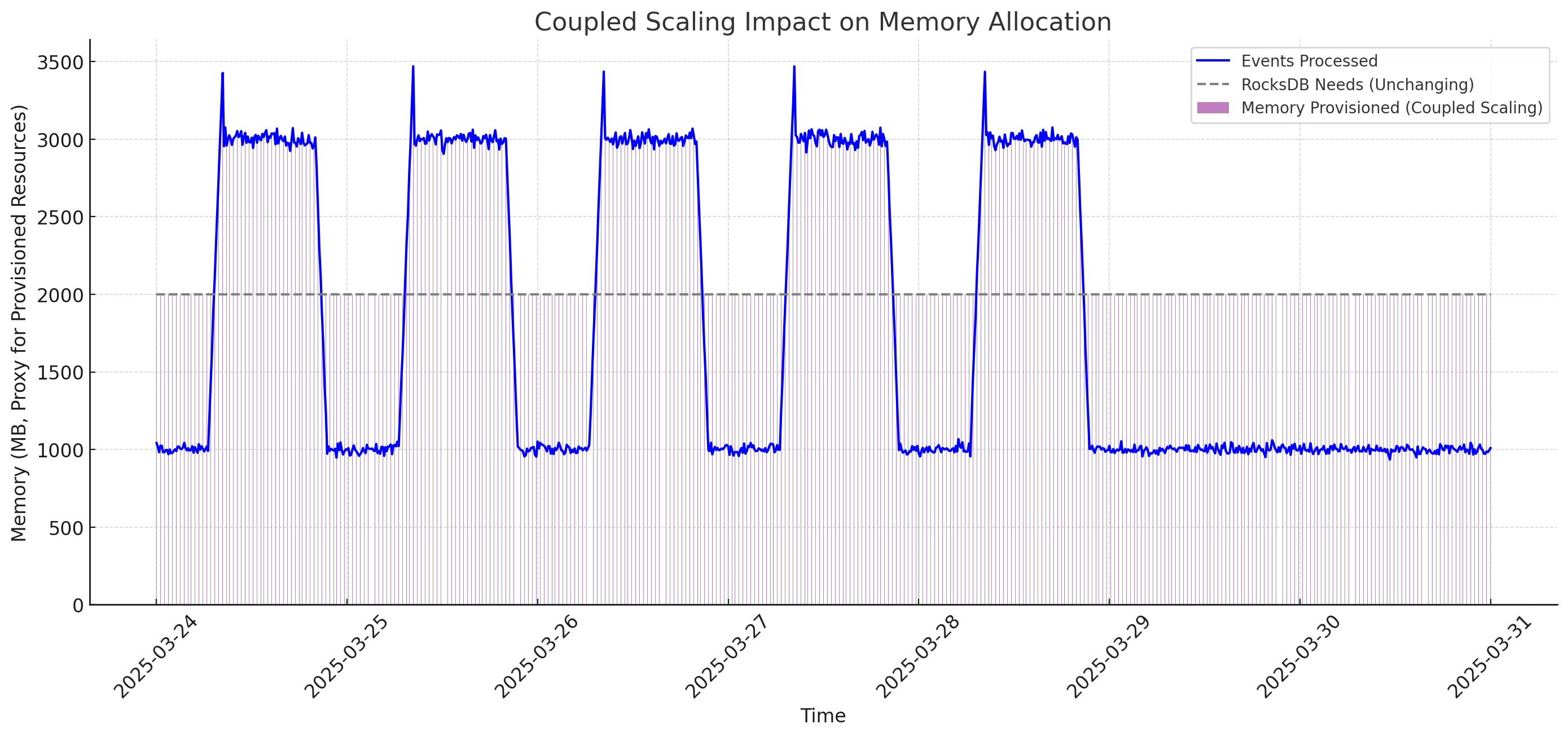
High Availability at a High Price
Let’s assume that Standby Replicas are a panacea for high availability in Kafka Streams (we’ve discussed before why they are not). Even then, they’re an expensive solution to the problem.
There are three costs that factor into standbys:
- The additional EBS storage. This one is obvious, to run your application with a single standby replica doubles your storage needs.
- Additional Memory/Compute. The calculator assumes 15% additional resources required, and this is likely to be conservative. RocksDB still needs to run the same amount of compactions, though you certainly need less cache because there are no lookups on standby replicas.
- More Kafka Egress. If you’re running with standbys, you are constantly reading the changelog topic from Kafka.
Somewhat surprisingly, in certain deployments of Kafka Streams (as is the case in the default example on https://www.kafkastreams.money) configuring a standby replica can cost more than your active processing cost.
With Responsive, You Pay Only for Active Processing
Let’s summarize each component of the cost calculator, and understand how Responsive cuts that cost:
Component | Cost (Rough %) | How Responsive Saves Money |
---|---|---|
Active Processing | 10-15% | N/A - this is the “real” work! |
Over Provisioning | 10-20% (depends on seasonality) | Autoscaling means you are always correctly provisioned. Separated compute/storage makes autoscaling possible. |
Standbys | 10-20% | Standbys aren’t required with Responsive at all since the storage backend is highly available. |
Storage | 15-25% | No storage is necessary for the Kafka Streams nodes. Instead, we provide cheaper storage via RS3 (see next section) |
RocksDB Compute | 20-30% | No rocksDB is necessary. Instead we provide efficient compute via RS3 (see next section) |
Restore Kafka Egress | 10-20% | No restores happen with Responsive, it’s part of our architecture. |
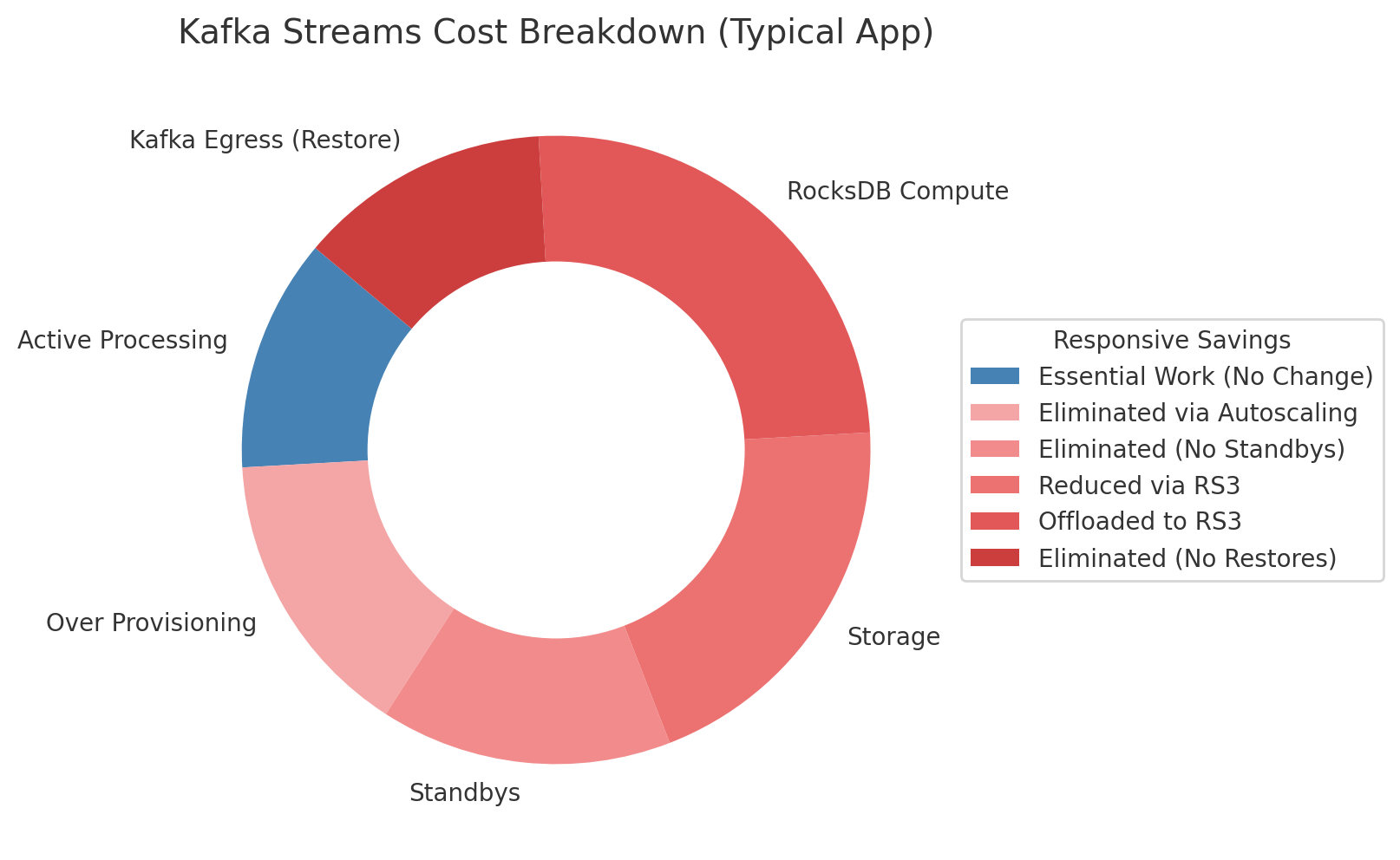
And all of this doesn’t start to consider the less quantifiable costs associated with open source Kafka Streams like the cost of lagging due to rebalances/restoration or the time spent by developers debugging incidents and shepherding deployments of stateful apps.
RS3 Clocks in Cheaper Than RocksDB
While Responsive completely eliminates some costs (like over-provisioning, Kafka egress from restoration and standby compute/storage), it does shift some costs over. Specifically, it shifts costs from RocksDB Compute and Storage to a dedicated database that is highly available in its own right.
When we launched Responsive, the options for the database were Cassandra (including implementations like Scylla) and MongoDB (Including implementations like CosmosDB, DocumentDB, etc.). Both of these options clock in at about 3x more expensive than the RocksDB component. In some deployments with those databases, Responsive still breaks even due to the cost components it eliminates but in many it was ultimately more expensive.
But today all that changes. Just as S3 changed the economics of distributed systems, RS3 changes the economics of storage for Stream Processing. It’s an object-store native database designed specifically to work with Kafka Streams and we’ve invested significantly into the underlying open source technology to make this a reality.
Honestly, it’s the most impressive piece of technology we’ve built to date.
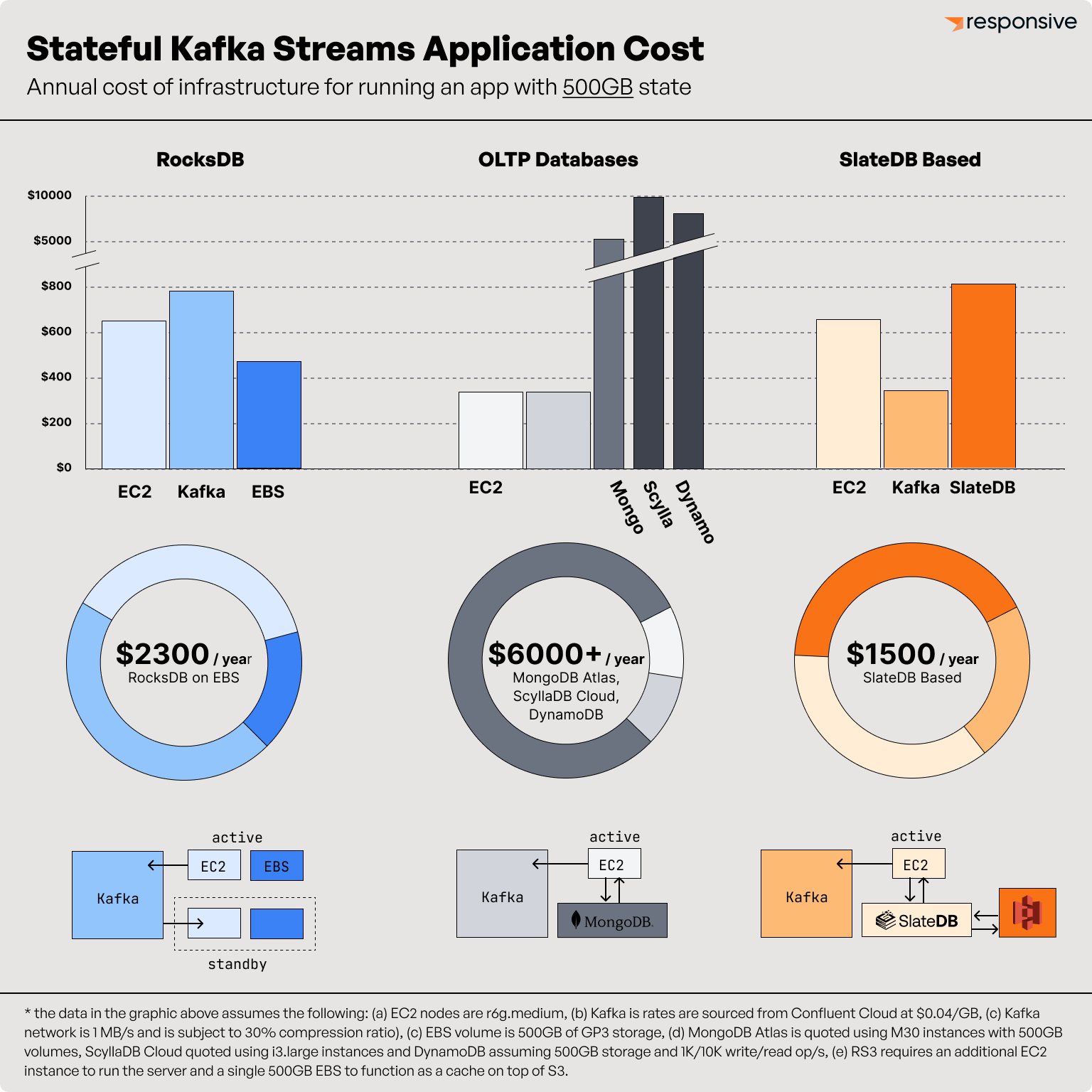
We discussed the economics behind it in more details in a previous post, but I’ll summarize the key takeaways here.
RS3 Slashes The Cost for HA
High availability and durability requires replication.
Typically, as is the case with Cassandra and MongoDB, replication is done over the network internally to a database and then the data is stored as copies on the local disk.
Kafka Streams pays an even higher cost for replication: it goes through Kafka. Every data that is written to RocksDB is written to a changelog topic, and then read from that changelog topic into the standby replica. The data is replicated 3 ways in the changelog topic, and then stored twice again on the Kafka Streams nodes as materialized state in RocksDB.
RS3, on the other hand, cheats the system by leveraging S3. The cost of replicating data in S3 is comically low — 1TB of highly replicated state costs $23/mo. The same data replicated 3x in different availability zones on EBS costs you $240/mo, and then an additional $20 of network costs to replicate it. If your data turns over 10 times a month, the data transfer costs of that replication costs you $200. S3, on the other hand, only charges you based on number of PUT and GET requests - which can be optimized to nearly 0.
This means the cost of maintaining replicated data on S3 is 1/20th of the cost of maintaining the same data in EBS (which is what other databases and RocksDB must do).
Now to be totally accurate, we also need to consider the fact that RS3 requires one replica on EBS for hot access - otherwise we’ll have a very inefficient system that frequently reads/writes to S3. But since this isn’t replicated cross-AZ, we don’t pay for the replication at all.
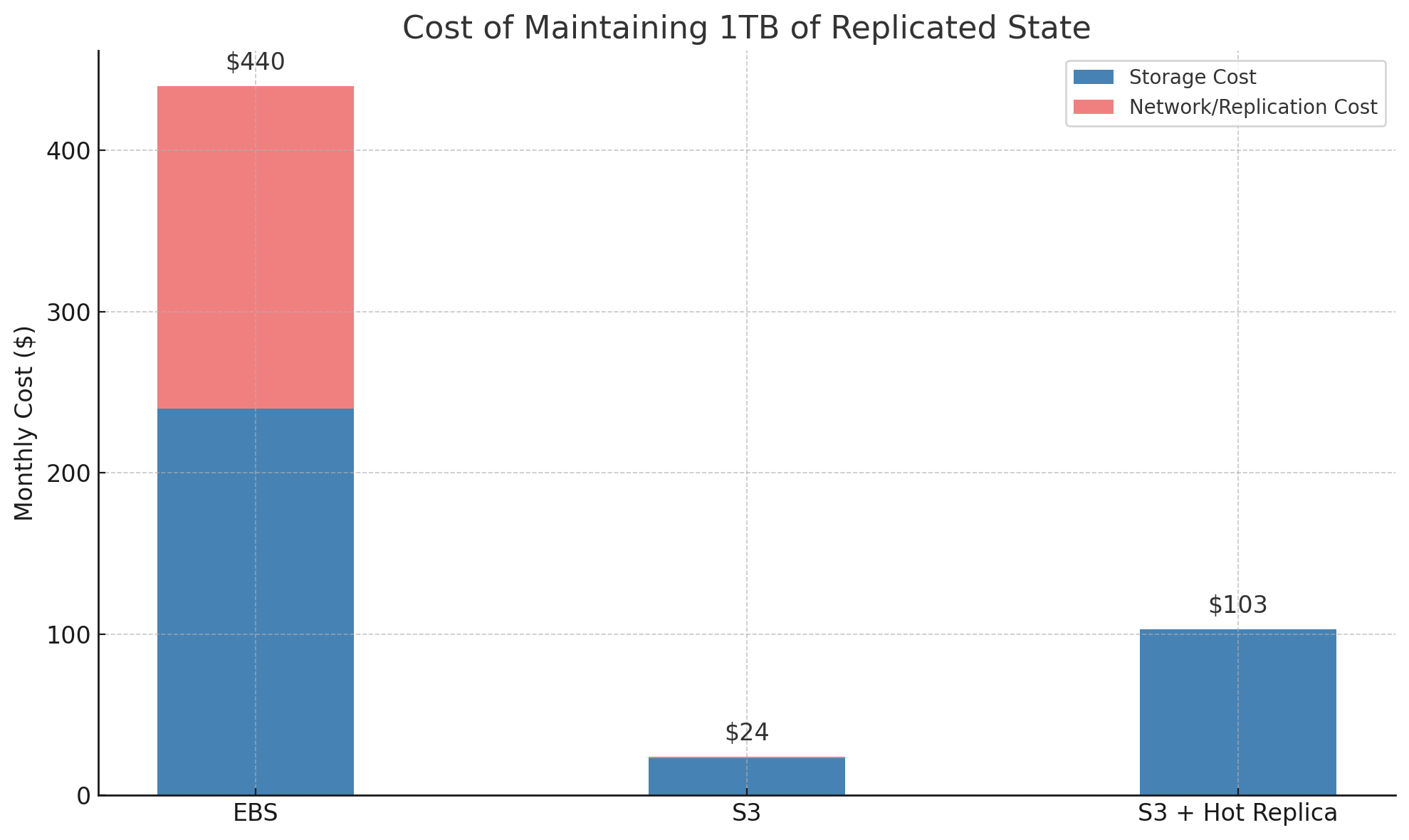
On-Demand Capacity for Compaction
The good thing about good architecture is that the gift keeps on giving. We designed RS3 so that compaction and garbage collection can be scheduled to run anywhere, unlike traditional databases that run these tasks on the same nodes that serve the traffic.
Not only is this a simplified architecture, it makes for much more efficient utilization of capacity. In RocksDB, you don’t know when a compaction will trigger. It may trigger exactly when you have your biggest burst of traffic to date — and right at that moment it’ll require significant CPU, Memory and Disk.
The implications are straightforward: you need to provision so that you have enough room to handle a compaction in the event that it happens. In our experience, for example, ScyllaDB requests that operate your cluster at no more than 60% disk utilization (though you can often push that to 70%) and that we typically must leave 20-30% CPU for compaction.
With RS3, all that unused capacity is unnecessary. You can schedule a compaction on a node when you need it, and leave your main serving capacity untouched.

We Don’t Charge a Mystical Compute Unit
By now, you’ve seen how Responsive can dramatically reduce your Kafka Streams infrastructure costs. But let’s talk about the other side of the equation: how much do we charge for it?
We believe the way stream processors are priced today is fundamentally broken—and we’ve built something better. Frankly, I think our pricing model is just as innovative as our core technology.
The Problem with “Compute Units”
Before founding Responsive, I spent years building KSQL at Confluent. One of the most frustrating questions I heard from customers was: "How much will it cost?" And I never had a good answer.
We priced KSQL in Confluent Streaming Units (CSUs)—a term that sounds more like a sci-fi currency than a real billing metric. What did a CSU actually buy you? No one could say. Because the truth is, the same machine might handle tens of thousands of events per second for a simple filter, and only a few hundred for a complex join. The only way to know was to deploy it and find out.
Sadly, this is common in our industry. Most Flink vendors charge this way, as does Databricks for Spark.
But here’s the catch: when vendors bill you based on abstract compute units, they’re usually just reselling cloud infrastructure—with a hefty markup. The more compute you use, the more you pay them. Which also means: the less efficient their framework is, the more money they make.
It’s a misalignment. And we wanted no part of it.
Origin Events + Number of Managed Nodes
To me, it’s clear that the future of Cloud technologies is BYOC — specifically customers demand having data in their own VPC since it dramatically simplifies the security and networking model.
We’ve fully embraced this at Responsive, and let you bring your own instances to run Kafka Streams. It’s so easy to operate a stateless Responsive instance that there’s no reason for us to run them on your behalf, instead we just manage them for you.
To make money of this management, we charge on two dimensions that are predictable and in your control:
Origin Events
We charge based on origin events—the raw input to your streaming system. These are the events that come directly from your applications or services before any joins, filters, or aggregates are applied. (See our docs to learn how we track them.)
We don’t charge you for derived events, intermediate streams, or the internal complexity of your application logic. That’s your business, not our billing model.
This gives you the freedom to design clean, composable topologies. Break your logic into small, maintainable applications. Split functionality across teams. Responsive doesn’t penalize good architecture.
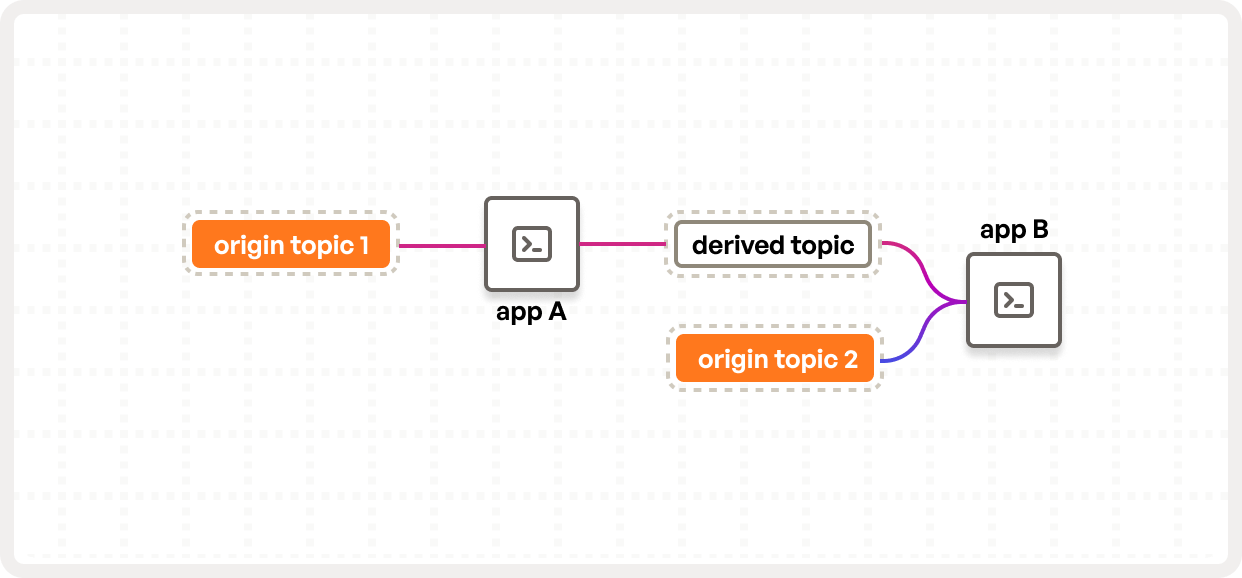
Number of Application Instances
We also charge a flat fee per application instance we manage. It doesn’t matter if you're running them on a high-memory, high-core container or a t3.micro—we charge the same. Why? Because the cost of managing a node is the same for us, no matter its size.
This model puts you in control. Want finer-grained autoscaling? Use smaller instances. Do you have predictable workloads and want to pay us less? Use bigger ones. Either way, the pricing is fixed, transparent, and predictable.
The Bottom Line (Fixing Your Top Line)
Kafka Streams isn’t broken—but the way we run it today is.
We’ve accepted slow restores, overprovisioned infrastructure, fragile standby replicas, and ballooning costs as the price of doing real-time work.
Responsive cuts through the complexity. It gives you Kafka Streams with better availability, better scalability, and lower costs—without compromising the API or the developer experience. You keep the power of Kafka Streams. You lose the baggage.
We’ve rethought storage with RS3. We’ve rethought pricing so it actually aligns with your success. And we’ve rethought the architecture so you don’t have to over-engineer around limitations that shouldn’t exist in 2025.
If you’ve been burned by the cost and operational overhead of stream processing, it’s time to try something better.
Run the calculator. — Try our product. — Or just talk to us.
You’ll wonder why you ever settled for less.
Want more great Kafka Streams content? Subscribe to our blog!
Almog Gavra
Co-Founder